AI in FP&A: What’s Real, What’s Hype, and What’s Next
- strategIQ Finance
- May 6
- 10 min read
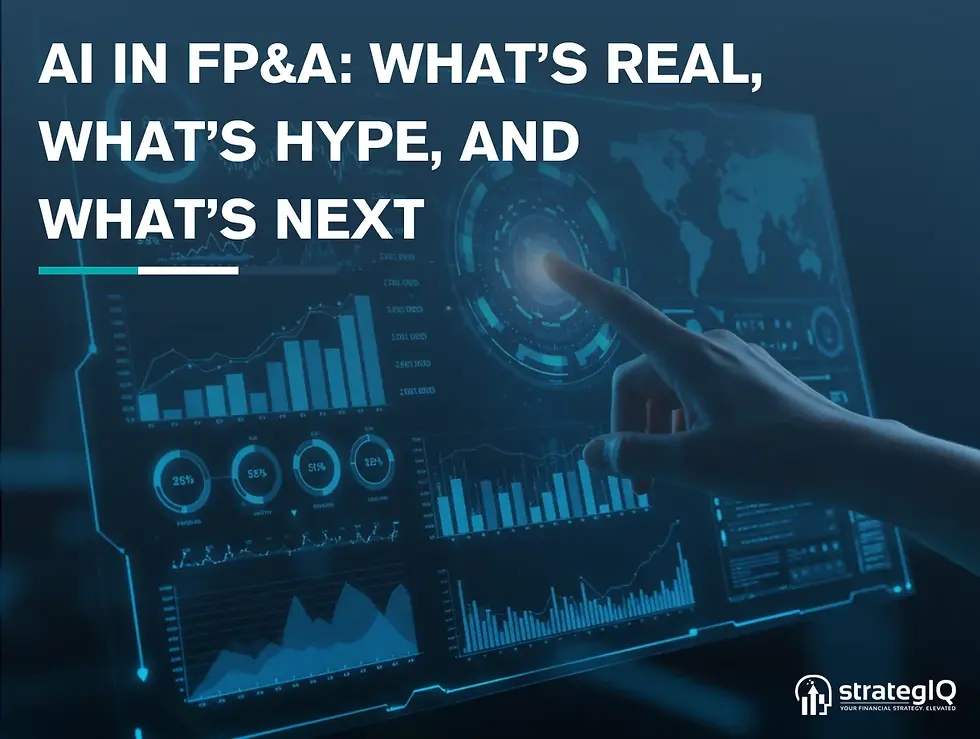
The intersection of artificial intelligence and financial planning and analysis (AI in FP&A) represents one of the most significant technological shifts in modern finance.
Furthermore, as financial leaders navigate this rapidly evolving landscape, distinguishing between practical applications and overblown promises becomes increasingly crucial.
Organizations that strategically implement AI in FP&A are gaining substantial competitive advantages, while those relying on outdated methodologies risk falling behind.
Understanding both the current capabilities and limitations has become essential for financial decision-makers aiming to maximize ROI on technology investments.
As we examine the real-world applications, we must consider how these technologies are reshaping financial roles and responsibilities.
Therefore, this comprehensive analysis explores what's genuinely transformative, what's merely hype, and what innovations lie on the horizon for AI in FP&A.
The Current State of AI in FP&A: Beyond the Buzzwords
Moving past the initial excitement, the current landscape of AI in FP&A reveals tangible applications and evolving capabilities. This section explores the real-world integration of AI within financial planning and analysis, offering a grounded perspective.
Defining AI, ML, and Predictive Analytics in Financial Contexts
Before diving deeper into applications, it's essential to establish clear definitions of what AI in FP&A actually encompasses. Primarily, artificial intelligence in financial contexts refers to systems capable of performing tasks that typically require human intelligence.
Consequently, when organizations implement AI in FP&A, they're typically deploying some combination of these technologies to enhance financial decision-making processes.
Moreover, understanding these distinctions helps financial leaders make more informed technology investment decisions rather than pursuing AI implementations based solely on industry buzz.
The AI Implementation Reality: Adoption Rates Among Financial Leaders
Despite widespread interest, the actual adoption of advanced AI in FP&A remains uneven across the financial sector.
According to a 2024 survey by Gartner, only 37% of finance organizations have moved beyond pilot projects to full AI implementation in their FP&A functions.
Many organizations continue to struggle with implementation challenges, including data quality issues, integration with legacy systems, and talent gaps.
Therefore, while AI in FP&A presents tremendous opportunities, a realistic assessment of organizational readiness remains critical for success.
Why Most AI Initiatives Fail in Finance Organizations
Despite significant investments, many AI initiatives fail to deliver expected returns. Primarily, McKinsey research shows that 70% of all AI projects fail to generate substantial business value.
Furthermore, within finance departments specifically, implementation failures often stem from several common factors.
Initially, many organizations underestimate the data preparation required, clean, consistent financial data forms the foundation for any successful initiative.
Inadequate change management leads to resistance among finance professionals who may view AI as threatening rather than augmenting their roles.
Understanding these potential pitfalls allows organizations to develop more realistic implementation roadmaps for AI in FP&A technologies.
Real-World Applications: Where AI is Actually Delivering Value
Beyond theoretical discussions, AI is actively generating value in specific FP&A functions, streamlining processes and enhancing insights. This section highlights concrete examples of successful AI deployments and the tangible benefits realized by organizations.
Automating Data Aggregation and Financial Close Processes
Specifically, AI-powered systems can extract data from disparate sources, reconcile inconsistencies, and prepare consolidated financial statements with minimal human intervention.
Furthermore, organizations implementing AI in FP&A for these purposes report reducing their financial close times by an average of 36%, according to research from Hackett Group.
Error rates in financial reporting decrease significantly when AI in FP&A tools handles routine data processing tasks. Consequently, finance professionals can redirect their efforts from manual data manipulation to higher-value analytical activities.
The consistency and reliability improvements from automated financial reporting provide a more solid foundation for subsequent financial analysis and decision-making.
Enhancing Forecast Accuracy with Machine Learning Models
AI in FP&A is revolutionizing forecasting accuracy through sophisticated machine learning models that identify patterns invisible to traditional methods.
Indeed, these systems analyze thousands of variables simultaneously, incorporating both internal financial data and external economic indicators to produce more reliable projections.
Machine learning models continuously improve as they process more data, leading to progressively more accurate forecasts over time.
AI-Powered Scenario Planning for Investment Decisions
Investment-focused organizations increasingly rely on AI to enhance scenario planning capabilities.
Primarily, machine learning algorithms can rapidly generate and evaluate thousands of potential scenarios based on varying market conditions, interest rates, and other economic factors.
Additionally, these tools enable investment analysts to quantify risks more precisely and identify optimal asset allocations across diverse market conditions.
Anomaly Detection and Risk Management
AI in FP&A excels at identifying unusual patterns that might indicate errors, fraud, or emerging business risks. Indeed, machine learning systems can establish baseline patterns of financial transactions and flag deviations that warrant further investigation.
The continuous monitoring capabilities of AI in FP&A systems provide an additional layer of security and compliance assurance for financial organizations.
The Hype vs. Reality: Setting Realistic Expectations
While AI offers significant potential for FP&A, it's crucial to establish realistic expectations regarding its current capabilities and limitations. This section aims to demystify the hype surrounding AI and provide a balanced perspective on its practical application.
Capabilities and Limitations of Current AI Solutions
While AI offers substantial benefits, understanding its current limitations is equally important. Primarily, today's AI systems excel at pattern recognition and prediction based on historical data but struggle with novel situations without precedent.
Additionally, many tools remain heavily dependent on the quality of data inputs; garbage in still means garbage out, regardless of algorithmic sophistication.
However, while AI can process vast datasets quickly, it lacks the contextual understanding and ethical judgment that experienced financial professionals provide.
Common Myths About AI in Financial Analysis
Several persistent myths continue to distort expectations around AI in FP&A. Initially, many executives believe that implementing AI will immediately transform their finance operations, overlooking the gradual learning curve these systems require.
Consequently, dispelling these myths allows organizations to develop more realistic implementation strategies for AI. Understanding the actual capabilities and limitations of these technologies enables more productive conversations about how AI can enhance strategic financial planning processes.
The True ROI Timeline for AI Implementation
Expectations regarding return on investment for AI in FP&A often need recalibration. Indeed, while some benefits like reduced manual processing may materialize quickly, the full value of predictive capabilities typically emerges over longer timeframes.
Furthermore, organizations that view AI implementation as a multi-stage journey rather than a one-time project generally report higher satisfaction with outcomes.
Implementation Blueprint for Investment-Focused Organizations
For investment-focused organizations looking to leverage AI in their FP&A functions, a strategic implementation blueprint is essential. This section outlines key considerations and steps for successfully integrating AI tools and workflows.
Essential AI Implementation Steps for PE Firms and Family Offices
For investment-focused organizations specifically, implementing AI in FP&A requires a structured approach. Initially, conducting a thorough assessment of current processes identifies the highest-value opportunities for AI enhancement.
Additionally, beginning with narrowly defined use cases, like automating portfolio company data collection or enhancing specific budgeting forecasting models, builds institutional confidence before expanding to more complex applications.
Data Requirements and Governance Considerations
Successful AI in FP&A implementation hinges on robust data management practices. Primarily, organizations must establish clear data governance frameworks defining ownership, quality standards, and access protocols for financial information.
Consequently, many organizations find they must upgrade their data infrastructure before advanced AI applications can deliver reliable insights.
Managing Security and Compliance Concerns
Financial organizations implementing AI in FP&A must address heightened security and compliance considerations. Indeed, AI systems often require access to sensitive financial data, necessitating robust protection mechanisms.
Additionally, regulatory frameworks like GDPR, CCPA, and industry-specific regulations impose specific requirements on how AI systems can utilize and store financial information.
Building vs. Buying: Strategic Approaches to AI Adoption
Organizations face critical decisions about whether to develop proprietary AI in FP&A solutions or implement commercial platforms.
Primarily, building custom solutions offers greater alignment with specific business processes but requires substantial technical expertise and ongoing maintenance resources.
Commercial platforms provide faster implementation and regular updates but may necessitate process adaptations to fit standardized functionality.
Lastly, many organizations find success with hybrid approaches that combine commercial foundations with customized elements for differentiating capabilities.
Strategic Advantages: AI as a Competitive Differentiator
Early adopters of AI in FP&A are beginning to realize significant strategic advantages, gaining enhanced analytical capabilities and improved decision-making. This section explores how AI can serve as a key competitive differentiator in the investment landscape.
Gaining Time Advantages in Deal Analysis
For investment organizations, AI delivers critical speed advantages in transaction assessment. Indeed, machine learning algorithms can analyze target company financials in hours rather than the days or weeks required by traditional methods.
Additionally, automated data processing eliminates bottlenecks in deal pipelines, allowing firms to evaluate more opportunities without proportional increases in analytical resources.
AI in FP&A tools can quickly surface red flags or highlight particularly promising aspects of potential investments. As a result, firms gain precious time advantages in competitive deal environments, potentially securing better terms through faster decision-making.
Enhanced Due Diligence Through AI-Powered Analytics
AI transforms the depth and breadth of due diligence capabilities available to investment professionals. Specifically, natural language processing can analyze thousands of contracts, agreements, and financial documents to identify potential liabilities or opportunities.
Similarly, machine learning models can detect unusual patterns in financial statements that might indicate accounting irregularities or undisclosed issues.
Furthermore, AI systems can compare target companies against thousands of similar transactions to identify valuation anomalies or overlooked synergy opportunities.
Portfolio Optimization and Real-Time Performance Monitoring
Post-acquisition, AI in FP&A continues delivering value through sophisticated portfolio monitoring capabilities. Primarily, these systems can track hundreds of performance indicators across portfolio companies, alerting investment teams to emerging issues or opportunities requiring attention.
Thus, investment managers can make more timely interventions when portfolio companies deviate from expected performance trajectories. Ultimately, the ability to analyze performance across multiple dimensions simultaneously provides a more nuanced understanding of value creation levers.
Cross-Fund Analytics and Pattern Recognition
Organizations managing multiple investment vehicles gain unique advantages from implementing AI in FP&A. Indeed, these systems can identify patterns across different funds, sectors, and investment stages that remain invisible when analyzed in isolation.
Additionally, machine learning models can detect which investment theses consistently outperform across varying market conditions.
Finally, AI technologies enable more sophisticated attribution and variance analysis, distinguishing between market-driven returns and value created through active management.
The Human-AI Partnership in Modern FP&A
The most effective integration of AI in FP&A involves a collaborative partnership between human professionals and intelligent technologies. This section examines how this synergy can optimize workflows and leverage the unique strengths of both.
Evolving Skill Requirements for FP&A Professionals
The integration of AI in FP&A is fundamentally reshaping skill requirements for finance professionals. Primarily, technical capabilities like data interpretation, statistical understanding, and basic programming knowledge have become increasingly valuable alongside traditional financial expertise.
Consequently, finance organizations are evolving their hiring and development practices to cultivate this multidisciplinary skill set.
Where Human Judgment Remains Critical
Despite advancing capabilities, numerous aspects of financial planning still require human judgment that AI cannot replicate. Indeed, evaluating qualitative factors like management team dynamics, corporate culture, or emerging market trends remains firmly in the human domain.
As a result, the most effective implementations of AI in FP&A create human-machine partnerships rather than replacement relationships.
Organizational Structure for AI-Enhanced Finance Teams
Implementing AI in FP&A often necessitates structural changes within finance organizations. Specifically, many leading firms establish centers of excellence that bridge financial expertise and data science capabilities.
Cross-functional teams incorporating IT, data management, and finance professionals help ensure AI systems remain aligned with business objectives. Furthermore, some organizations create new roles like "Finance Technology Translators," specifically tasked with bridging technical and financial domains.
What's Next: The Future of AI in Financial Planning
The field of AI in financial planning is rapidly evolving, with new advancements and applications on the horizon. This section explores potential future trends and the transformative impact they may have on the FP&A function.
Emerging AI Technologies on the Horizon
Several emerging technologies promise to further transform AI capabilities in the coming years. Primarily, large language model applications are beginning to enable natural language interfaces between finance professionals and complex financial data.
Moreover, establishing technology scanning processes helps organizations prepare for emerging capabilities before they become competitive necessities.
From Reactive to Predictive to Prescriptive Analytics
The evolution of AI follows a clear progression from explaining what happened to anticipating what will happen to recommending optimal actions.
Indeed, while many organizations have implemented predictive capabilities, truly prescriptive systems that autonomously recommend specific financial decisions remain relatively rare.
The Integration of Alternative Data Sources
Future AI in FP&A applications will increasingly incorporate non-traditional data sources to enhance predictive accuracy. Specifically, satellite imagery, social media sentiment, supply chain data, and Internet of Things inputs offer leading indicators that complement traditional financial metrics.
Moreover, evaluating the marginal predictive value of different data sources helps prioritize integration efforts.
Preparing Your Organization for the Next Wave of AI Innovation
Forward-thinking finance organizations are already laying the groundwork for next-generation AI in FP&A capabilities.
Consequently, organizations that view AI as a continuous journey rather than a destination position themselves for sustainable competitive advantage.
Moreover, balancing immediate operational needs with longer-term capability development remains essential for capturing the full potential of artificial intelligence in financial planning and analysis.
Conclusion
The integration of AI represents both a tremendous opportunity and a significant challenge for today's financial organizations. Indeed, distinguishing between genuine value creation and temporary hype requires a clear understanding of current capabilities and limitations.
Additionally, successful implementation demands thoughtful approaches to technology selection, data management, and organizational change.
Consequently, investment-focused firms that strategically deploy these technologies gain substantial competitive advantages through enhanced decision speed, analytical depth, and forecasting accuracy.
Therefore, by understanding what's real, what's hype, and what's next in AI in FP&A, financial leaders can chart strategic paths toward technology-enhanced performance while avoiding costly implementation pitfalls. StrategIQ will assist you with our insights on this.
FAQs on AI in FP&A
1) Will AI replace FP&A professionals in investment management?
While AI will augment FP&A in investment management by automating tasks and enhancing analysis, human professionals' strategic thinking and judgment remain crucial. AI will likely assist, not replace, their higher-value activities.
2) How much does it typically cost to implement AI in an FP&A function?
The cost of AI implementation in FP&A varies based on complexity and integration needs, potentially including software, fees, and specialized talent. Long-term ROI through efficiency and better decisions should be considered.
3) What specific AI tools are most effective for private equity financial analysis?
Effective AI tools for private equity include NLP for due diligence, ML for performance prediction, and AI-powered data visualization. The specific tool depends on the firm's analytical requirements.
4) How can family offices ensure data security when implementing AI solutions?
Family offices ensure AI data security by choosing reputable vendors with strong protocols, implementing strict access controls and encryption, and adhering to data privacy regulations. Thorough due diligence and ongoing monitoring are also essential.
5) What are the minimum data requirements needed to successfully implement AI in FP&A?
Successful AI in FP&A generally requires a substantial volume of clean, well-structured historical financial data, along with relevant non-financial data. Data quality and consistency are often more critical than just quantity.
6) How long does it typically take to see ROI from AI implementations in finance?
ROI from AI in finance can range from one to three years or longer, depending on project scale and the benefits measured. A phased approach and clear KPI tracking are crucial.
7) Which FP&A processes should be prioritized first for AI enhancement?
FP&A processes to prioritize for AI enhancement are often repetitive, data-intensive tasks like data collection and variance analysis. Processes with high potential for improved accuracy, such as forecasting, are also good starting points.
8) How can we measure the success of our AI implementation in financial planning?
Success of AI in financial planning can be measured by improved forecast accuracy, reduced time on routine tasks, and new insights leading to better decisions. User adoption and feedback are also important indicators.
Comments